
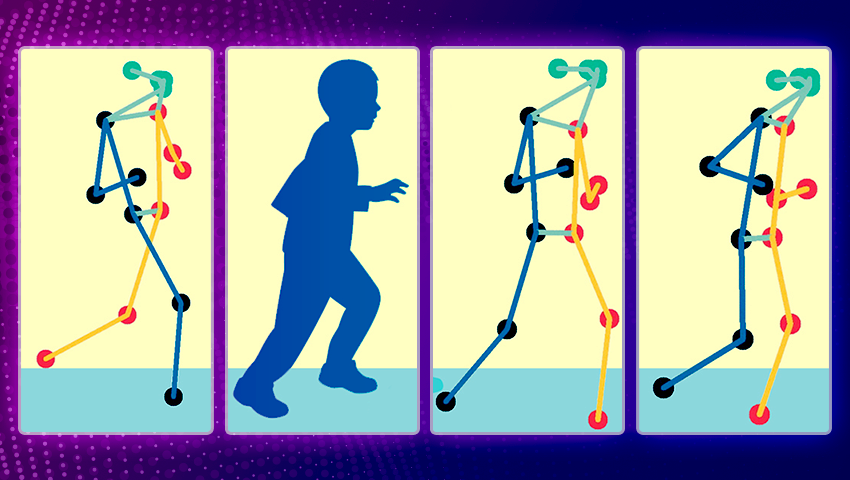
In the realm of medical diagnostics, innovation knows no bounds. Recently, an exciting breakthrough has emerged at the intersection of computer vision and machine learning, promising to revolutionize the way we assess and evaluate patients, particularly those with motor disorders such as cerebral palsy. This groundbreaking development, known as the Pose-Mapping Techniqueis set to reshape the landscape of medical diagnosis and patient care.
Traditionally, evaluating patients’ motor function, especially for conditions like cerebral palsy, necessitates frequent in-person visits to the doctor’s office. This process can be not only cumbersome but also financially burdensome and emotionally taxing, particularly for children and their parents. However, thanks to this cutting-edge technique developed by MIT engineers, we’re now on the cusp of a transformative leap forward in medical assessment.
At the heart of this innovation lies the seamless integration of computer vision and machine learning. By harnessing these advanced technologies, the Pose-Mapping Technique has unlocked the potential to remotely assess patients’ motor function. The method analyzes real-time videos of patients, deciphering specific patterns of poses within these videos. This analysis, driven by machine learning algorithms, computes a clinical score of motor function.
To develop a technique for analyzing skeleton pose data in patients with cerebral palsy, a condition typically assessed using the Gross Motor Function Classification System (GMFCS), scientists employ a five-level scale representing a child’s overall motor function (lower numbers indicate higher mobility).
The team used a publicly available set of skeleton pose data provided by Stanford University’s Neuromuscular Biomechanics Laboratory. This dataset contained videos featuring over 1,000 children with cerebral palsy, each demonstrating various exercises in a clinical environment. Additionally, each video was tagged with a GMFCS score assigned by a clinician following an in-person assessment. The Stanford group processed these videos through a pose estimation algorithm to generate skeleton pose data, serving as the foundation for MIT’s subsequent study.
Remarkably, the Pose-Mapping Technique exhibited an accuracy rate exceeding 70% that matches the assessments of clinicians during in-person evaluations. This level of precision holds immense promise for streamlining patient assessments and reducing the need for frequent and arduous trips to medical facilities.
The potential applications of the Pose-Mapping Technique extend far beyond cerebral palsy. The research team is currently tailoring the approach to evaluate children with metachromatic leukodystrophy, a rare genetic disorder affecting the nervous system. Furthermore, they are actively working on adapting the method to assess patients who have experienced a stroke.
Hermano Krebs, a principal research scientist at MIT’s Department of Mechanical Engineering, envisions a future where patients can reduce their reliance on hospital visits for evaluations. “We think this technology could potentially be used to remotely evaluate any condition that affects motor behavior,” he says. This implies a seismic shift in the way we approach medical assessments and patient care.
The road to this remarkable achievement began with computer vision and algorithms designed to estimate human movements. Pose estimation algorithms paved the way for the translation of video sequences into skeleton poses. These poses, represented as lines and dots, were then mapped to coordinates for further analysis.
The research team leveraged a Spatial-Temporal Graph Convolutional Neural Network to decipher patterns in cerebral palsy data, classifying patients’ mobility levels. Astonishingly, training the network on a broader dataset, which included videos of healthy adults performing daily activities, significantly enhanced its accuracy in classifying cerebral palsy patients.
The true marvel of this innovation is its accessibility. The method can be seamlessly executed on a multitude of mobile devices, ensuring widespread availability and real-time processing of videos. The MIT team is actively developing an app that could empower patients to take control of their self-assessments. This app would allow parents and patients to record videos within the comfort of their homes. The results could then be shared effortlessly with healthcare professionals, paving the way for more informed and timely interventions. Moreover, the method’s adaptability extends to evaluating other neurological disorders, promising to reduce healthcare costs and enhance patient care.
As was mentioned, the integration of computer vision and machine learning is rapidly transforming the landscape of medical diagnostics. At QuData, we share this enthusiasm for innovative solutions in medical imaging, contributing to a brighter future for healthcare. Our case studies provide a closer look at our ML research and solutions. Read more about our latest project Breast Cancer Computer-Aided Detection – AI-driven solution for enhancing breast cancer diagnosis precision and efficiency.